In 2024, a study by Washington University School of Medicine and tech startup Whiterabbit.ai found that AI may be able to detect early cases of breast cancer, as well as reduce false positives.
The company trained an AI model on 12,248 2D digital mammograms (6,161 showing cancer). They then tested the model against several real-world mammograms.
The study found that out of the 10,000 people who had mammograms, over 260 of them could have avoided diagnostic exams and 10 could have avoided biopsies using AI systems to help diagnose the presence of cancer.
Generative AI and large language models (LLMs) are rapidly transforming the healthcare landscape as we know it, offering innovative solutions to longstanding challenges. This paradigm-shifting technology has the potential to revolutionize patient care, streamline operations, and improve medical research.
This is the future of our healthcare system, and it's only the beginning.
In this comprehensive article, we'll dive deep into this new and exciting frontier and explore how ethical generative AI integration can aid in quality healthcare delivery and patient care.
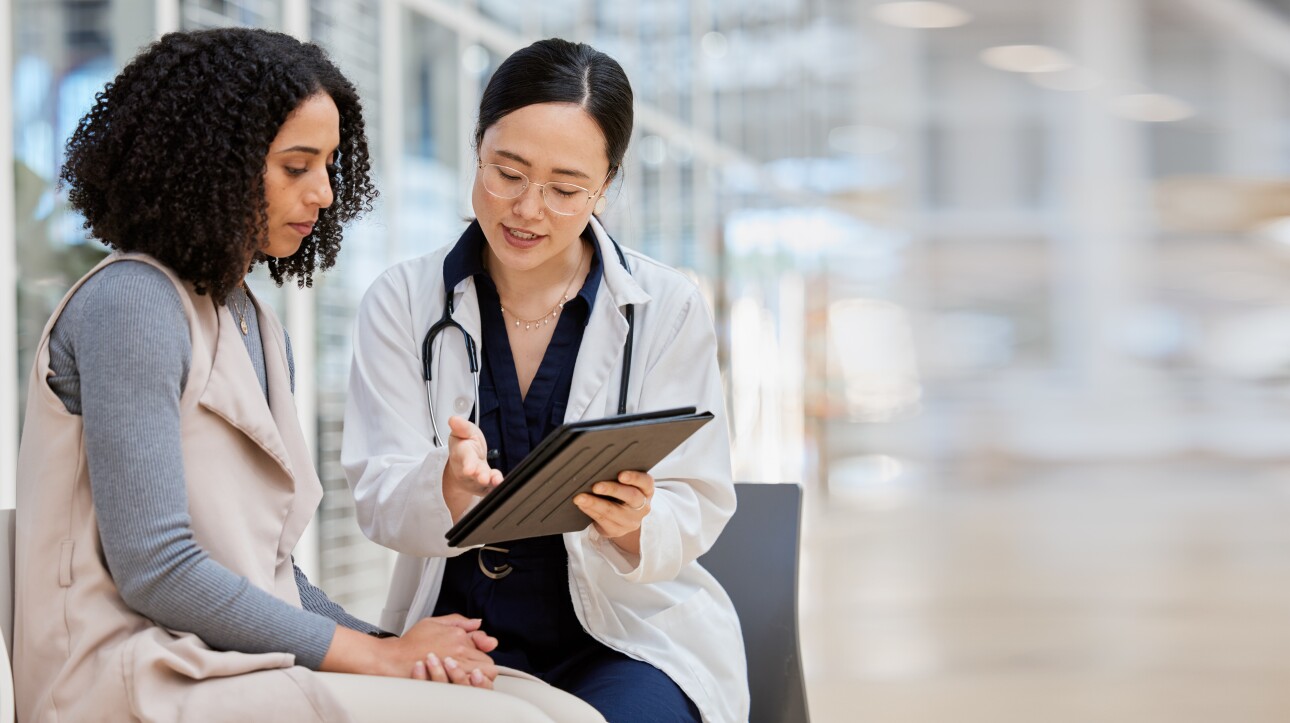
Key Takeaways
- Generative AI has the potential to revolutionize healthcare through automation and improved clinical applications.
- Healthcare leaders are actively adopting generative AI tools to improve patient experiences and streamline operations.
- Generative AI integration in healthcare presents opportunities for improved outcomes but also raises ethical and privacy concerns. Every organization needs to consider these in their journey to adopting generative AI.
What is Generative AI in healthcare?
You've likely seen how GPT-4 (often known by its more popular chat interface, ChatGPT) has disrupted industries worldwide. You may have even seen generative AI models like Flux and Midjourney create AI portraits that are almost indistinguishable from real-life photographs.
But this technology isn't just for creating art or generating intelligent text responses for corporate chat bots.
Generative AI in healthcare can automate administrative tasks, improve clinical applications, and ultimately lead to better patient outcomes.
Healthcare leaders are actively pursuing or planning to implement generative AI tools to enhance patient experiences and improve their operations.
As the healthcare industry embraces generative artificial intelligence, it faces both opportunities and challenges. While the technology promises to improve efficiency and accuracy in many areas, concerns about data privacy, ethical considerations, and regulatory compliance must be addressed.
Large language models (LLMs) have existed for decades, they've only recently burst into the mainstream. This chain reaction of popularity was most certainly ignited by OpenAI's landmark release of ChatGPT in late 2022.
That means the impact these technologies can have on healthcare remains poorly understood or misguided.
What's Special About Generative AI in Healthcare?
Traditionally, the healthcare industry has been relatively slow to adopt AI, except for specific areas such as radiology.
Because healthcare is a regulated industry with life-and-death decisions, it's appropriate that changes are made in a careful and measured way.
Healthcare is experiencing a crisis-level labor shortage. Doctors, nurses, and staff are chronically overworked, still recovering from the pandemic, and stressed with the amount of administration and paperwork required by law.
Clinicians often talk about "pajama time" – time spent after work hours updating charts, keeping up with patient email, and doing administration. These tedious (but necessary) tasks in healthcare are especially well-suited to generative AI technologies.
So even with its high levels of regulation, this industry is one of the top adopters of generative AI and is eager for even more AI applications – so long as they’re implemented ethically, safely, and sustainably.
Foundations of Generative AI in Healthcare

Generative AI isn't a "ghost in the machine" composing new information out of thin air. Rather, it uses vast datasets to create new content, help in diagnosis, and enhance treatment planning.
AI Technologies in Medicine
AI technologies in medicine encompass a wide range of applications.
Here's just a sampling of what's possible:
- Machine learning algorithms can analyze patient records to predict disease risks and recommend personalized treatments.
- Computer vision systems interpret medical imaging, helping radiologists in detecting abnormalities.
- Natural language processing (NLP) extracts valuable information from clinical notes and medical literature.
- Robotics and AI combine to improve surgical precision and automate repetitive tasks.
- Virtual and augmented reality powered by AI provide immersive training experiences for medical students and professionals.
- AI-driven chatbots and virtual assistants improve patient engagement and provide round-the-clock support for basic health queries.
- AI-powered agents and applications automate much of the routine work in record-keeping and patient correspondence for clinicians,
And much, much more. Let's explore some of these applications in more depth.
Applications of Generative AI
According to a survey we conducted at one of our healthcare events, many organizations believe generative AI is a powerful, but challenging technology with an almost infinite number of use cases.
These include innovative applications in diagnosis, treatment, personalized care, and drug development.
Diagnosis and Treatment Enhancement
Generative AI models are revolutionizing medical imaging analysis, improving diagnostic accuracy and speed. AI algorithms can detect subtle abnormalities in X-rays, MRIs, and CT scans that human eyes might miss.
In radiology, AI assists in identifying early signs of diseases like cancer or cardiovascular conditions. This leads to earlier interventions and improved patient prognoses.
Natural language processing capabilities enable AI to analyze vast amounts of medical literature and patient records. This helps healthcare providers make more informed treatment decisions based on the latest research and similar case histories.
AI-powered systems can also generate treatment plans tailored to individual patients. These plans consider factors like genetic makeup, medical history, and lifestyle to optimize therapeutic approaches.
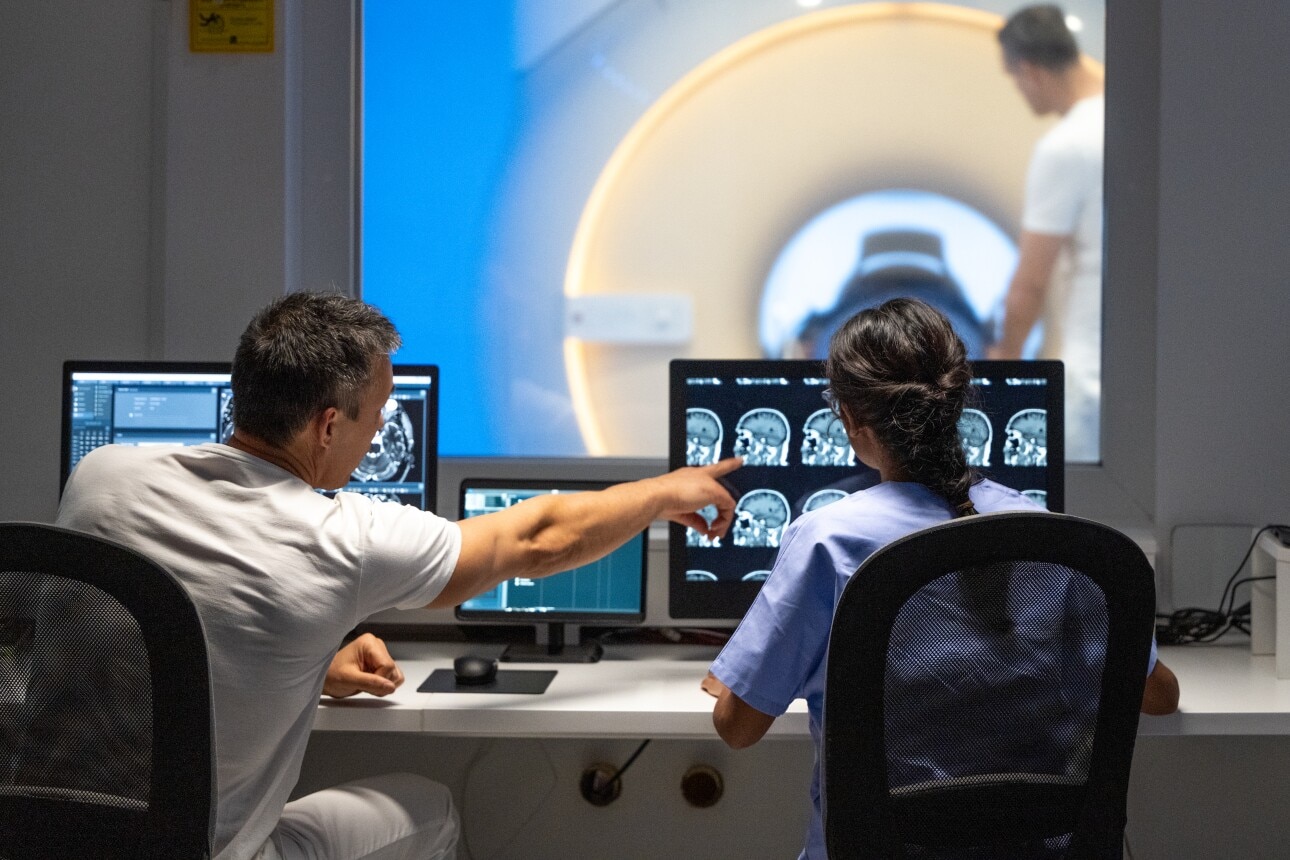
Personalized Patient Care
Generative AI is enhancing personalized care by analyzing patient data to predict health risks and recommend preventive measures. AI models can process diverse data types, including genetic information, to create individualized health profiles.
Chatbots and virtual assistants powered by generative AI provide 24/7 support to patients. They answer questions, offer medication reminders, and provide general health advice.
AI algorithms can generate personalized nutrition and exercise plans based on a patient's health status, goals, and preferences. This tailored approach improves adherence to healthy lifestyle recommendations.
In mental health care, AI-powered tools can analyze speech patterns and text to detect early signs of conditions like depression or anxiety. This enables timely interventions and support.
Drug Discovery and Development
Generative AI is accelerating drug discovery processes by predicting potential drug candidates and their interactions with biological targets. AI models can generate and screen millions of molecular structures, significantly reducing the time and cost of early-stage drug development.
Machine learning algorithms analyze vast datasets of chemical compounds, biological pathways, and clinical trial results. This helps researchers identify promising drug candidates and predict their efficacy and potential side effects.
AI-powered simulations can model drug interactions within the human body, allowing researchers to optimize dosages and formulations before clinical trials begin. This improves the success rate of drug development and reduces risks for trial participants.
In clinical trials, generative AI aids in patient selection and monitoring. It can identify suitable candidates based on specific criteria and predict potential adverse reactions, enhancing trial safety and efficiency.
Integrating AI with Healthcare Data
A big benefit of generative AI is that it can process a lot of data and find solutions that humans would be unable to find.
Integration between healthcare data and generative AI models is the key to improving patient care, streamlining workflows, and uncovering valuable insights from complex datasets.
Electronic Health Records (EHRs)
EHRs serve as a cornerstone for AI integration in healthcare. These digital records contain comprehensive patient information, including medical histories, lab results, and treatment plans.
AI algorithms can look at EHRs to find patterns and trends (including text and image data) that may not be clear to human doctors right away. This analysis can help predict potential health risks and suggest personalized treatment options.
Healthcare organizations are increasingly adopting AI-powered tools to extract meaningful information from unstructured EHR data. These tools can automatically categorize and summarize clinical notes, making it easier for medical professionals to quickly access relevant patient information.
Most EHR vendors, realizing the significance of the current AI gold rush, are hurriedly adding gen-AI-powered features to their systems. These allow for the following benefits:
- Easier navigation
- Hands-free interaction (voice recordings of patient interactions are mined for content and formatted)
- Better insight in clinical decision-making
AI-powered startups for healthcare were also one of the top categories for startup funding in 2024.
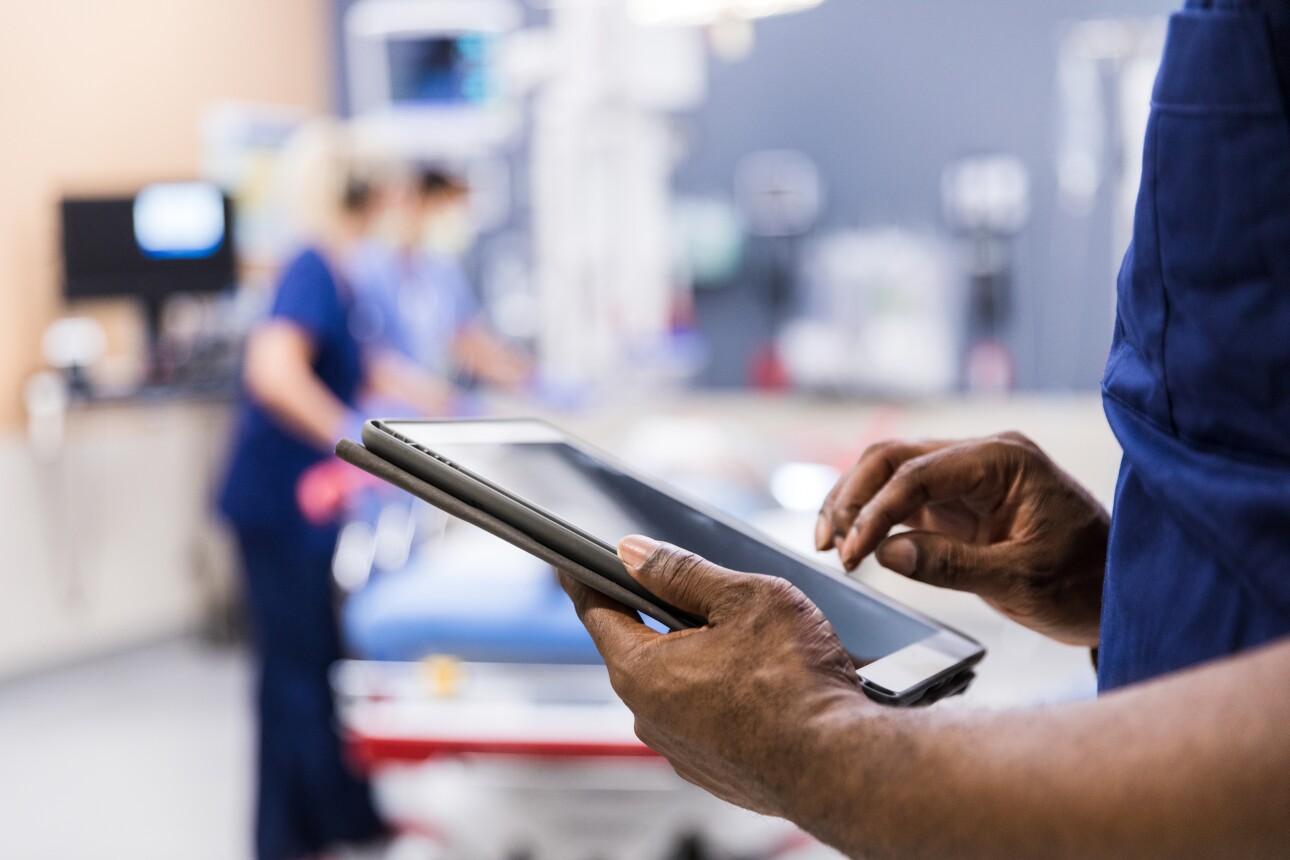
Data Analytics and Patterns
AI excels at processing large volumes of healthcare data to uncover patterns and make predictions. Advanced analytics techniques can identify correlations between various health factors, leading to improved diagnostics and treatment strategies.
Machine learning algorithms can analyze diverse datasets, including:
- Patient demographics
- Medical histories
- Lab results
- Imaging studies
- Genetic information
By examining these datasets, AI systems can detect subtle patterns that may indicate early signs of diseases or predict patient outcomes. This capability enables healthcare providers to implement proactive interventions and personalized treatment plans.
AI-driven predictive analytics also help healthcare organizations optimize resource allocation and improve operational efficiency. These tools can forecast patient admissions, identify high-risk patients, and suggest preventive measures to reduce hospital readmissions.
Ethics, Privacy, and Regulation
Generative AI in healthcare raises critical concerns around fairness, data protection, and governance. Addressing these issues is essential to ensure responsible implementation and maintain public trust in AI-powered medical technologies.
Addressing Bias and Equity
AI systems can perpetuate existing biases in healthcare data and practices. To mitigate this, developers must use diverse and representative training datasets. Regular audits of AI outputs help identify and correct biases.
Ethical AI development requires multidisciplinary teams including ethicists, clinicians, and patient advocates. This ensures AI tools consider varying perspectives and needs.
Equity in AI access is crucial. Healthcare providers should implement AI solutions that serve diverse patient populations, not just those with the most resources.
Patient Privacy and Data Security
Protecting patient privacy is paramount when using AI in healthcare. Strict data anonymization and encryption protocols must be enforced.
AI systems often require large datasets for training and operation. Healthcare organizations must implement robust cybersecurity measures to prevent data breaches.
Informed consent processes need updating to account for AI use. Patients should understand how their data may be used in AI systems and have the right to opt-out.
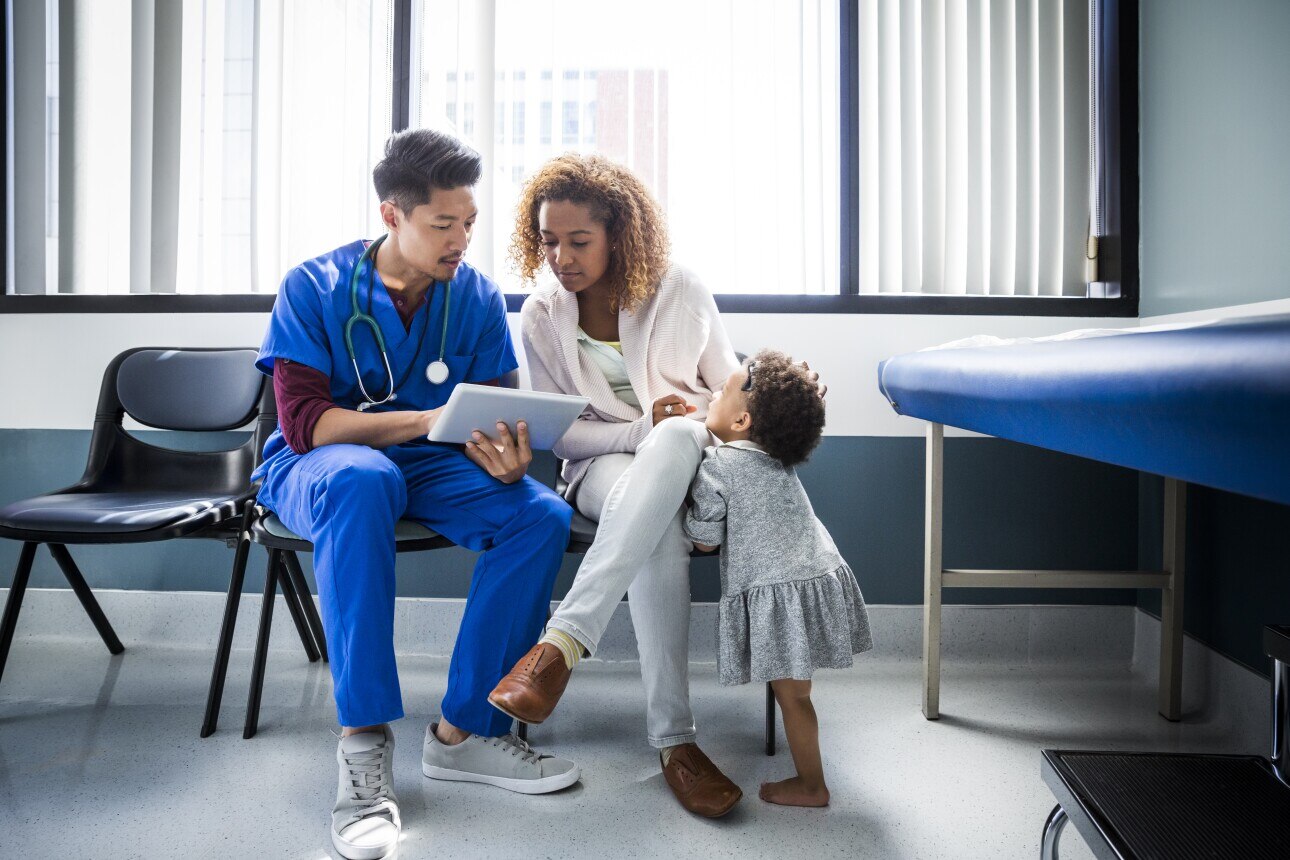
Regulatory Considerations
Governments worldwide are wrestling with how to best regulate AI. The FDA is developing frameworks to evaluate and manage AI-based medical devices. This includes guidelines for continuous monitoring and updating of AI algorithms.
The EU AI Act proposes strict rules for high-risk AI applications, including many in healthcare. It emphasizes transparency, human oversight, and accountability.
Liability issues in AI-assisted healthcare decisions remain complex. Clear guidelines are needed to determine responsibility when AI contributes to medical errors.
International cooperation is essential to create harmonized AI regulations. This ensures consistent standards and facilitates global development and deployment of healthcare AI.
Beyond governmental regulation, there are also multiple industry consortia focused on appropriate use of AI in healthcare, notably CHAI (Coalition for Health AI) and TRAIN (Trustworthy & Responsible AI Network).
Healthcare organizations are quickly adding AI to their rules and policies. Many of them are creating a Chief AI officer role or adding this to the Chief Data Officer's duties.
Challenges and Limitations
Generative AI in healthcare faces significant hurdles in technical capabilities and market acceptance. These challenges impact both the technology's effectiveness and its adoption in medical settings.
AI Hallucinations
Many high-stakes industries have been hesitant to implement generative AI models like GPT-4 and others for one glaring reason: AI hallucinations.
AI hallucinations happen when models generate false or nonsensical data, which obviously poses serious risks in medical contexts.
As models continue to improve, these hallucinations will become less and less prevalent, and there are ways to mitigate hallucinations with programmatic guardrails, such as:
- Fact-checking mechanisms: implementing systems that cross-reference AI-generated information with verified medical databases. Sometimes these are called "grounding" mechanisms.
- Human-in-the-loop approaches: ensuring that AI outputs are reviewed by healthcare professionals before being used in patient care.
- Confidence thresholds: only accepting AI-generated content when the model's confidence level exceeds a predetermined threshold.
- Multi-model consensus: using multiple AI models and only accepting outputs where there's agreement among them.
The Case for a "Slow Roll" Approach
Given these limitations, it may be ideal to "slow roll" generative AI applications in health systems, beginning with lower-risk applications such as:
- Administrative tasks: using AI for scheduling appointments, managing patient records, and handling billing inquiries.
- Patient education: generating personalized educational materials about conditions and treatments.
- Triage chatbots: implementing AI-powered chatbots to guide patients to appropriate care levels without making diagnoses.
- Research assistance: using AI to summarize medical literature and identify potential areas for further study.
- Medical coding: employing AI to assist in accurate and efficient medical coding for billing purposes.
By starting with these lower-risk applications, healthcare systems can:
- Build confidence in AI systems
- Develop robust protocols for AI implementation
- Gradually train staff on AI integration
- Identify and address potential issues before moving to higher-risk applications
As trust and capabilities grow, AI can be incrementally introduced into more critical areas of healthcare, always maintaining a strong focus on patient safety and ethical considerations.

Many of these lower-risk applications also provide major benefits to healthcare systems, so this slow-roll approach isn't truly a compromise.
By aiming at improving the clinician experience and reducing "pajama time," organizations can use a lower-risk, human-in-the-loop approach and simultaneously deliver major benefits.
Market and Adoption Barriers
Healthcare institutions face obstacles in integrating generative AI into existing systems. High implementation costs and the need for specialized infrastructure discourage many organizations from adopting these technologies.
Regulatory uncertainties surrounding AI in healthcare create hesitation among potential adopters, and concerns about liability and accountability for AI-driven decisions slow market growth.
Healthcare professionals may resist AI adoption due to fears of job displacement or loss of autonomy in decision-making. This resistance can significantly impede the integration of generative AI into clinical workflows.
Patient trust is another critical factor. Many individuals remain skeptical about AI involvement in their healthcare, preferring human interaction and judgment over machine-generated insights.
The Future of AI in Healthcare
Artificial intelligence is poised to revolutionize healthcare through predictive modeling and strategic collaborations. These developments promise to improve patient care, streamline operations, and accelerate medical research.
Predictive Models and Prognostics
AI-powered predictive models are set to transform healthcare decision-making. These tools will analyze vast amounts of patient data to forecast health outcomes and identify potential risks. For example, AI platforms may predict the likelihood of hospital readmissions or disease progression.
Machine learning algorithms will continue to improve, enabling more accurate diagnoses and personalized treatment plans. AI tools will help in interpreting medical imaging, potentially detecting diseases earlier than human clinicians.
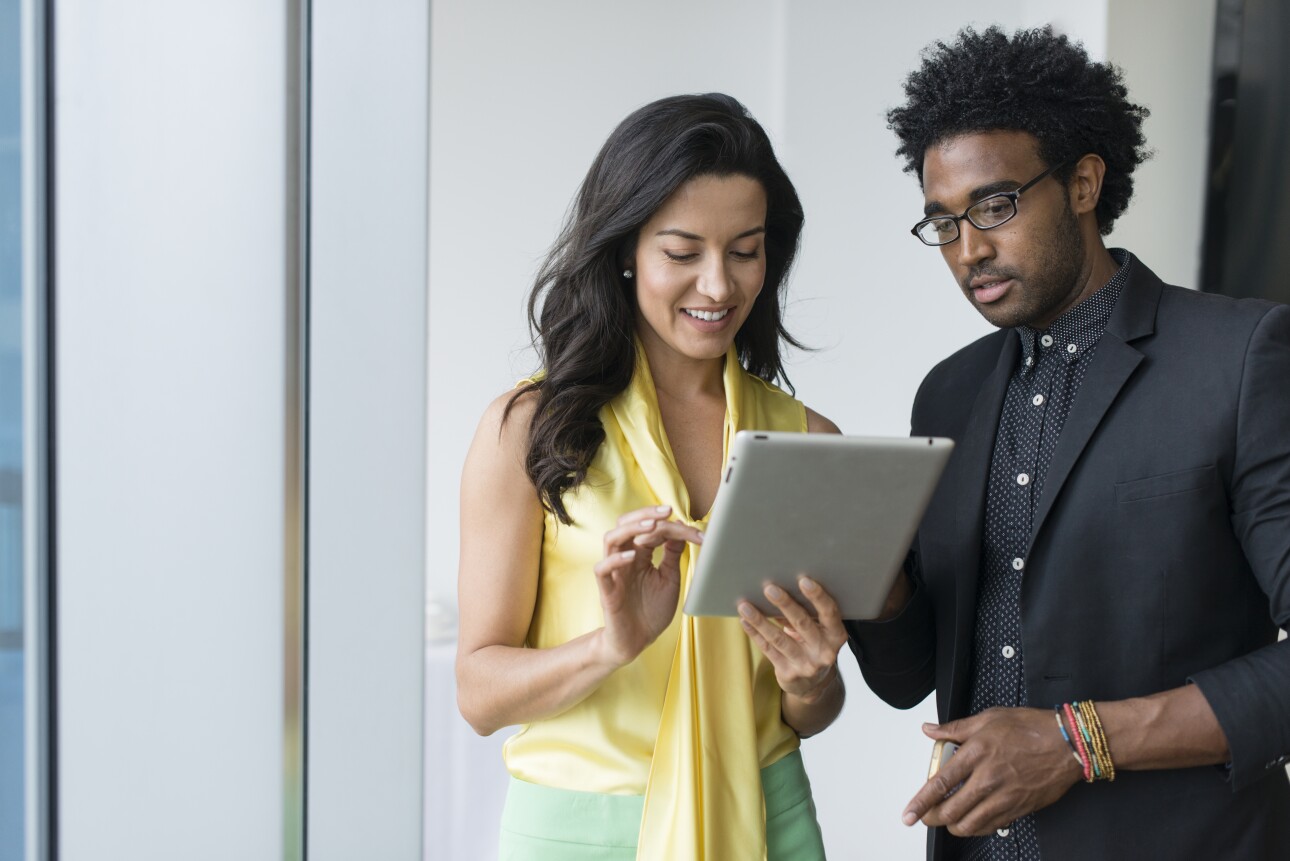
Strategic Partnerships and Collaboration
Healthcare organizations are forming strategic partnerships with tech companies to leverage AI expertise. These collaborations aim to develop innovative AI solutions tailored to healthcare needs.
OpenAI and similar organizations are working with medical institutions to create specialized AI models for healthcare applications. These partnerships focus on developing AI that can understand complex medical terminology and assist in clinical decision-making.
Startups are emerging with AI-powered mobile apps designed for both patients and healthcare professionals. These apps may offer symptom checkers, medication reminders, and virtual health assistants.
Large pharmaceutical companies are collaborating with AI firms to accelerate drug discovery and development processes. AI tools are being used to analyze molecular structures and predict drug efficacy, reducing the time and cost of bringing new treatments to market.
Generative AI and Mental Health
Generative AI has applications for both the body and the mind. LLMs have the potential of revolutionizing mental healthcare by providing innovative tools for diagnosis, treatment, and patient support.
This technology offers promising solutions to enhance therapeutic interventions and streamline mental health practices.
Supporting Mental Health Practices
Generative AI models are being developed to assist in diagnosing mental health conditions. These models analyze patient data, including verbal responses and behavioral patterns, to provide clinicians with valuable insights.
A study published in Nature suggests a three-step approach using generative AI for recognizing, expressing, and managing emotions.
One key insight is the potential for AI to create personalized visual aids, helping patients, especially children, to better identify and express their emotions. For example, AI could generate custom images depicting various emotional states, making abstract concepts more tangible and relatable.
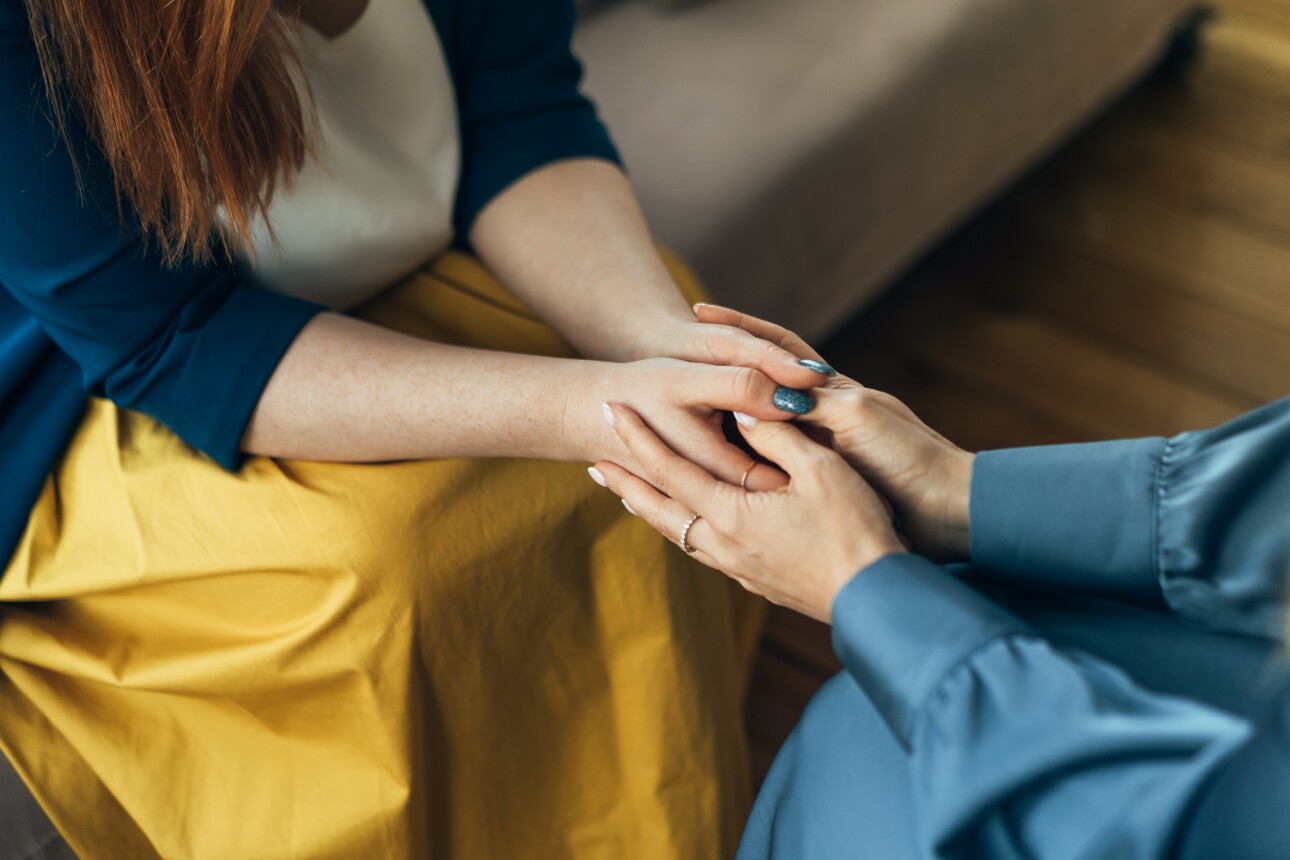
The study also emphasizes the importance of cultural sensitivity in AI-generated content, recognizing that emotional expression can vary significantly across cultures. This underscores the need for diverse and inclusive training data for AI models used in mental health applications.
Researchers propose that GAI could augment existing evidence-based therapies like Cognitive Behavioral Therapy (CBT), Dialectical Behavior Therapy (DBT), and Acceptance and Commitment Therapy (ACT) by providing tailored visual tools and personalized content.
As the field evolves, there's potential for integrating GAI with other technologies like virtual and augmented reality, opening up possibilities for more immersive and personalized therapeutic experiences.
Final Thoughts
The integration of generative AI in healthcare is ushering in a new era of patient care and diagnosis, with platforms like InterSystems IRIS playing a pivotal role in this transformation.
As we explore the full potential of AI technologies, it's clear that robust data management and integration solutions are essential for success.
By providing seamless interoperability, advanced analytics, and strong security features, InterSystems IRIS empowers healthcare organizations to harness the full potential of AI while ensuring data privacy and regulatory compliance.
Learn more about how InterSystems IRIS can help you ethically integrate generative AI into your healthcare organization by contacting us today.
Frequently Asked Questions
AI-powered chatbots are improving patient engagement and providing 24/7 support for basic health inquiries. These systems can automate administrative workflows, freeing up healthcare professionals to focus on complex patient care tasks.
Regulatory compliance and liability issues pose challenges. Healthcare organizations must navigate complex regulations to ensure AI systems meet safety and efficacy standards.
AI models can simulate clinical trials, helping researchers identify potential side effects and optimize dosing regimens before human testing begins.
Healthcare organizations must carefully manage patient consent and data access. Transparent AI systems that explain their decision-making process can help build trust with patients and providers.
Advanced predictive models could enable early disease detection and personalized risk assessments based on genetic and lifestyle factors.
AI-driven systems can adapt treatment plans in real-time based on patient responses and new medical research. This dynamic approach may lead to better outcomes and reduced side effects.