RAG vs Fine-tuning vs Prompt Engineering
Retrieval Augmented Generation (RAG), fine-tuning, and prompt engineering are three of the most popular ways to train AI models for particular business use cases.
Each method offers distinct advantages, and choosing the right approach, or combination of approaches, can significantly impact your AI application's success.
This article breaks down each approach, examining their strengths, limitations, and ideal use cases. We'll explore when to use each method, how to implement them effectively, and how InterSystems IRIS can support your chosen strategy.
Whether you're just starting with AI enhancement or looking to optimize existing applications, this guide will help you make informed decisions about your AI implementation approach.
Criteria | Prompt Engineering | RAG | Fine-tuning |
Implementation | Easy | Medium | Complex |
Cost | Low | Medium | High |
Accuracy | Variable | High | High |
Maintenance | Low | Medium | High |
Quick Summary of the Differences
Each method offers unique advantages for improving large language model (LLM) performance:
- Prompt Engineering: The basic approach of crafting specific instructions to guide language model responses
- RAG (Retrieval Augmented Generation): Enhances LLM outputs by connecting to external knowledge sources
- Fine-tuning: Adapts pre-trained models for specific tasks through additional training
These three approaches serve different needs and can be used independently or together. Prompt engineering offers the fastest path to implementation, making it ideal for initial AI projects and testing.
RAG adds reliability by connecting AI responses to verified information sources, which helps prevent incorrect outputs and keeps responses current.
Fine-tuning requires more upfront work but can create highly specialized AI models that perform consistently for specific tasks. Many successful AI implementations combine multiple approaches - for example, using RAG to provide accurate information while leveraging fine-tuning to maintain consistent response formats.
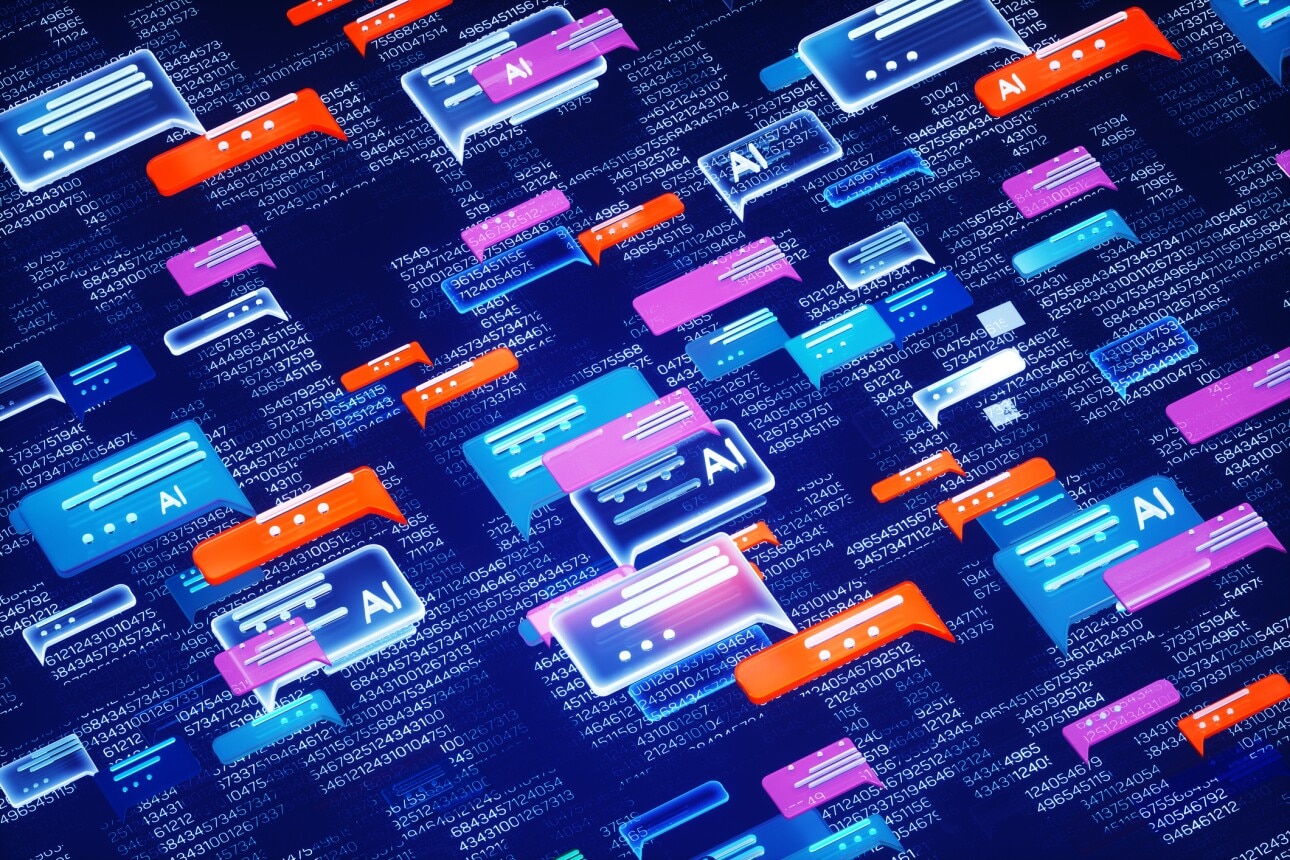
Prompt Engineering Fundamentals
Prompt engineering offers the fastest path to implementation, making it perfect for initial AI projects.
What It Is and How It Works
Prompt engineering involves creating clear instructions for LLMs to generate desired outputs. It's the foundation of effective AI interaction, requiring careful attention to wording and structure.
Key Components
Effective prompt engineering relies on several essential components working together.
1. Clear Instructions
At its foundation are clear instructions that tell the LLM exactly what you want it to do. These instructions should be specific and unambiguous, avoiding vague directions that could lead to inconsistent results.
2. Context Setting
Context setting provides the LLM with background information about its role and purpose. For example, you might specify that the LLM should act as a technical support specialist with expertise in database systems, or indicate that it should write in a specific tone for your target audience.
3. Examples
Examples, often called few-shot learning, show the LLM what good outputs look like. By providing 2-3 high-quality examples of questions and answers, you help the model understand the patterns it should follow. This approach is particularly effective when you need specific formatting or consistent response styles.
4. Output format specifications
Output format specifications tell the LLM exactly how to structure its response. This might include requirements for JSON formatting, specific headers, or particular ways of organizing information. Clear format guidelines ensure that the LLM's outputs can be easily processed by other parts of your application.
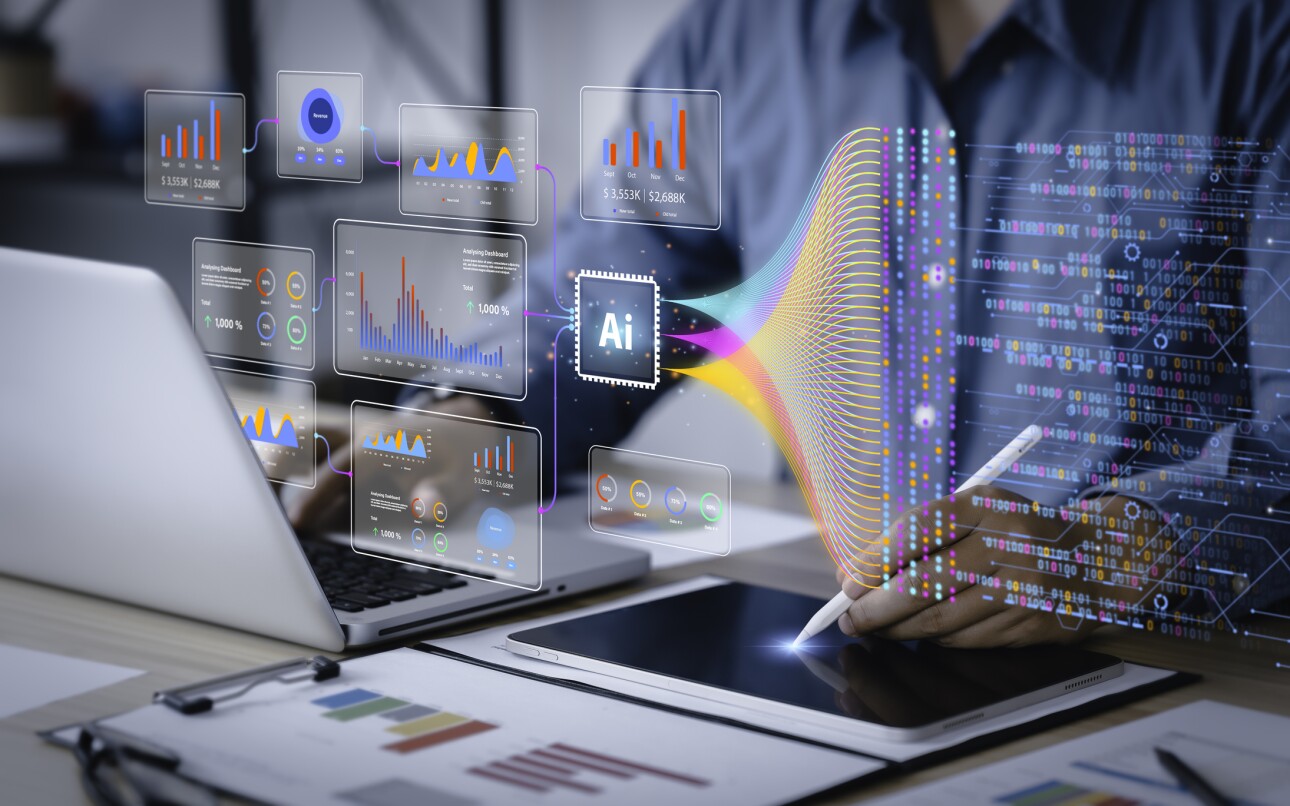
Advantages and Limitations
Advantages:
- Simple to implement: Creating prompts requires only basic writing skills and understanding of LLMs. Anyone can start crafting prompts with minimal technical background.
- No additional infrastructure needed: You can begin using prompt engineering with just an API key and access to an LLM service. There's no need for databases, servers, or complex technical setups.
- Quick to modify and test: Changes to prompts can be made instantly and tested immediately with real queries. This rapid iteration allows for quick refinement of your AI application's responses.
- Cost-effective starting point: Since you only pay for API usage without additional infrastructure costs, prompt engineering offers a practical way to start AI projects with minimal investment.
Limitations:
- Limited by context window size: Each LLM has a maximum number of tokens it can process at once. This means you can't include large amounts of information or long conversations in a single prompt.
- Requires expertise in prompt crafting: While getting started is easy, creating consistently effective prompts takes practice and deep understanding of how LLMs interpret instructions. Small changes in wording can significantly impact results.
- May produce inconsistent results: Without tight controls, the same prompt might generate different responses each time. This variability can make it difficult to maintain consistent output quality.
- Cannot add new knowledge to the model: The model can only work with information from its original training data. Any new facts or updates must be included in each prompt, making it inefficient for applications requiring a lot of current or specialized knowledge.
When to Use Prompt Engineering
Choose prompt engineering if you can answer YES to:
- Can your task be explained clearly in a prompt?
- Is general knowledge sufficient for your needs?
- Are you comfortable with some variation in responses?
- Do you need a solution running quickly?
- Is your budget limited?
If you answer NO to two or more of these questions, consider exploring RAG or fine-tuning approaches instead.
Red Flags
Prompt engineering might NOT be the best choice if:
- You need to reference large amounts of specific information on which the LLM was not trained.
- Your application requires perfectly consistent outputs
- You're handling sensitive or confidential data
- You need real-time or current information
- Your application will handle thousands of requests per hour
- You need complex, multi-step reasoning with high accuracy
Retrieval Augmented Generation (RAG)
RAG combines the power of LLMs with real-time data access, making it ideal for applications requiring current information.
How RAG Works
RAG combines LLMs with external data sources, allowing real-time access to information not included in the original training. This makes it especially useful for applications requiring current or specialized knowledge.
System Components
1. Knowledge Base or Document Store
At the core of any RAG system is its knowledge base, which houses all the information the system can access. This component stores your organization's documents, articles, manuals, and other text-based resources. The quality and organization of this information directly impacts the accuracy of your system's responses.
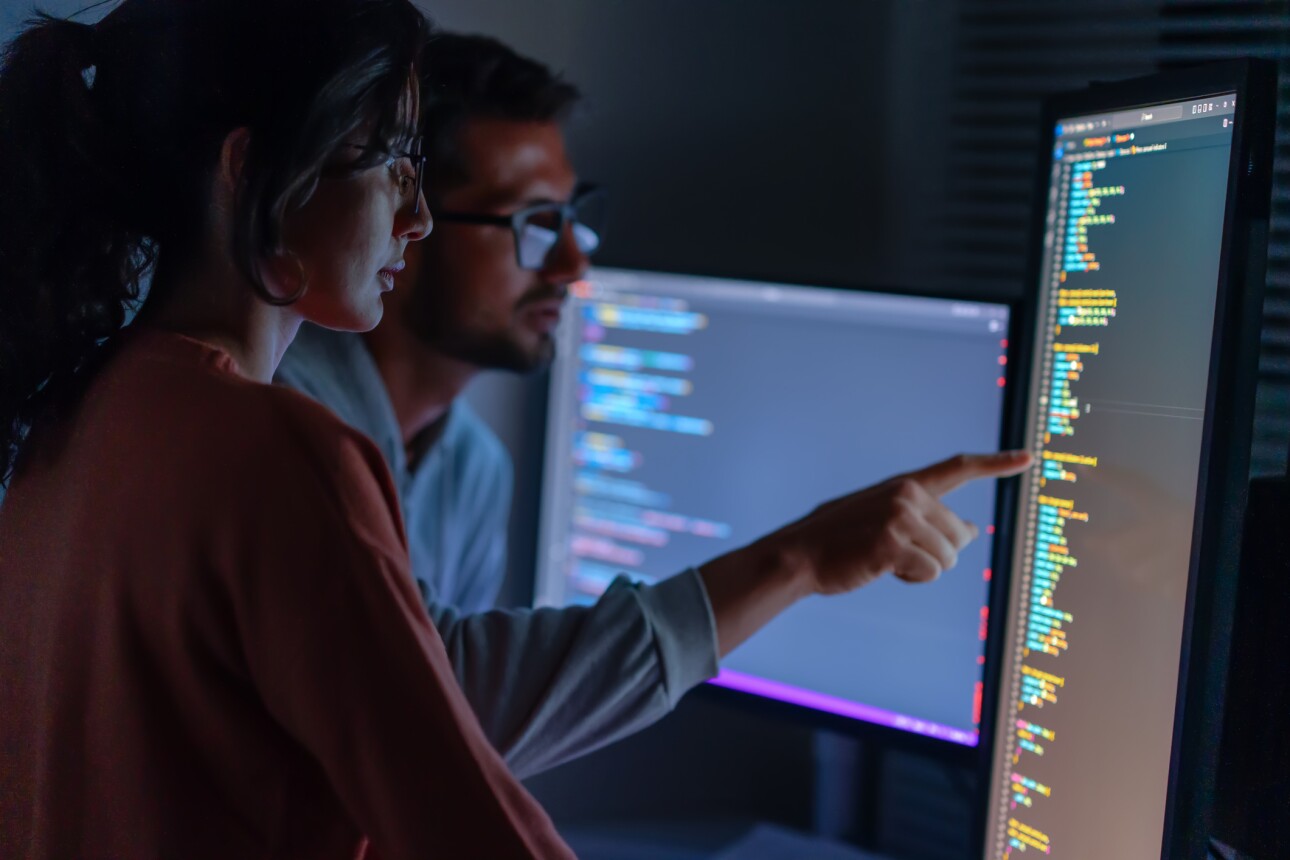
2. Vector Database
The vector database serves as the intelligent search engine of your RAG system. Unlike traditional databases that match exact words, vector databases understand the meaning behind the text. They store information in a mathematical format that allows for quick similarity searches, making it possible to find relevant information even when the wording differs from the original query.
3. Embedding Model
The embedding model acts as a translator, converting human language into a format that computers can efficiently process. It takes text - both from your stored documents and incoming queries - and transforms it into numerical vectors that capture the meaning of the content. These vectors enable the system to understand relationships and similarities between different pieces of text, making semantic search possible.
4. Retrieval System
The retrieval system works as the coordinator, managing how information flows between components. When a question comes in, this system processes it through the embedding model, searches the vector database, and ensures the retrieved data is relevant before passing it to the LLM.
5. Large Language Model
The LLM functions as the expert communicator, receiving both the user's question and the retrieved relevant information. It processes this combined input to generate natural, coherent responses that incorporate the retrieved knowledge. The LLM ensures that responses are not only accurate based on the retrieved information but also well-structured and easy to understand.
Benefits and Challenges
Benefits:
- Access to up-to-date information: Your LLM can reference and use the latest information from your knowledge base, making it perfect for applications that need current data like product details or company policies.
- Reduced hallucinations: By grounding responses in actual documents and data, RAG significantly decreases the likelihood of the LLM making up incorrect information.
- Verifiable responses: Every answer can be traced back to specific sources in your knowledge base, making it easier to validate the accuracy of responses and build trust with users.
- Scalable knowledge base: Your system can grow with your needs as you add new documents and information without requiring model retraining.
Challenges:
- More complex implementation: Setting up a RAG system requires multiple components working together, making it more technically challenging than simple prompt engineering.
- Additional processing time: The need to search for and retrieve relevant information adds extra steps to each query, potentially increasing response times compared to direct LLM calls.
- Data management overhead: Keeping your knowledge base current, properly formatted, and well-organized requires ongoing effort and careful attention to data quality.
When to Use RAG
Choose RAG if you can answer YES to:
- Do you need to reference specific documents or data sources?
- Is factual accuracy critical for your application?
- Does your knowledge base update frequently?
- Do you need verifiable sources for responses?
- Are you working with domain-specific or proprietary information?
- Can you invest in proper infrastructure setup?
If you answer NO to two or more of these questions, consider using simple prompt engineering or exploring fine-tuning instead.
Red Flags
RAG might NOT be the best choice if:
- Your information fits easily within standard prompt lengths
- You can't dedicate resources to maintaining a knowledge base
- Your use case requires instant responses with minimal latency
- You lack technical resources for setup and maintenance
- Your primary need is consistent formatting rather than accurate information
- Your budget can't support the necessary infrastructure
- You need offline functionality without database access
Fine-tuning Deep Dive
Fine-tuning isn't about teaching new facts - it's about teaching new behaviors.
Process Overview
Fine-tuning adjusts a pre-trained model's parameters using specific data to improve performance on targeted tasks. This creates a more specialized model aligned with particular requirements.
How it Works
Fine-tuning builds upon an existing AI model's capabilities, similar to teaching a skilled professional a new specialty. The process starts with pre-trained large language models that already understand language and has broad knowledge. This base model serves as the foundation, much like a general education serves as the foundation for specialized training.
The actual fine-tuning process begins with collecting examples that show exactly what you want the model to learn. These examples come in pairs - an input (what you might ask the model) and an output (how you want it to respond). Quality matters more than quantity here - a few hundred well-crafted examples often work better than thousands of mediocre ones.

As you begin to fine tune, the model begins adjusting its internal connections based on these examples. Instead of learning language from scratch, it's learning your specific patterns and preferences.
The process uses a technique called "low-rank adaptation" (LoRA), which is remarkably efficient. Instead of modifying all of the model's parameters - which would be like rewriting an entire book - LoRA adjusts a small, strategic set of connections. This approach saves time and computing resources while still achieving excellent results.
During training, the model repeatedly processes your examples, gradually improving its ability to generate responses that match your desired style or format. It's testing itself constantly - trying to predict the correct outputs for your inputs, checking its answers against your examples, and adjusting its approach based on where it makes mistakes.
The process requires careful monitoring to prevent "overfitting" - where the model becomes too focused on your specific examples and loses its ability to handle new, slightly different situations. This is like making sure a student learns general principles rather than just memorizing specific answers.
Once fine-tuning is complete, you have a specialized version of the original model that maintains its broad capabilities but now excels at your specific task. This new model will need fewer detailed instructions in its prompts because the behavior you want has been built into its parameters. However, fine-tuning doesn't add new factual knowledge - it primarily teaches the model new patterns of behavior, formatting, or specialized ways of responding.
When to Use Fine-tuning
Choose fine-tuning if you can answer YES to:
- Do you need highly consistent output formatting or style?
- Are you processing a large volume of similar requests?
- Can you create high-quality training examples?
- Will you use this model for an extended period?
- Do you have access to machine learning expertise?
- Is reducing prompt length and inference costs important?
If you answer NO to two or more of these questions, consider using prompt engineering or RAG instead.
Red Flags
Fine-tuning might NOT be the best choice if:
- Your use case changes frequently or requires constant updates
- You can't create at least 50-100 high-quality training examples
- You need to reference current or real-time information
- Your budget can't support initial training costs
- You need the solution implemented within days
- You lack technical resources for model maintenance (fine-tuning can often be resource intensive)
- Your task requirements aren't clearly defined yet
- You need transparent, source-based responses
Ideal Scenarios
Fine-tuning works best when:
- Creating consistent customer service responses
- Generating standardized documents (reports, emails, summaries)
- Converting data into specific formats
- Writing in a particular brand voice or style
- Processing high volumes of similar requests
- Implementing specific business rules or policies
- Reducing operational costs for repetitive tasks
How InterSystems IRIS Can Power Your AI Enhancement Strategy
Choosing between prompt engineering, RAG, and fine-tuning doesn't have to be a complex decision. InterSystems IRIS provides you with the flexibility to implement any of these approaches—or combine them—based on your specific needs and goals.
What sets InterSystems IRIS apart is its comprehensive support for all three AI enhancement methods within a single platform. You don't need to piece together multiple systems or worry about complex integrations. Whether you're starting with simple prompt engineering or building sophisticated RAG systems, InterSystems IRIS provides the foundation you need.
Try InterSystems IRIS today and discover how your organization can leverage these AI enhancement approaches effectively, with the support of a platform that understands and adapts to your evolving needs.