Reconcile and Link Person Records Across Disparate Systems for AI and Beyond

The Importance of Accurate Identity Data
In health and care, getting identity right isn’t just important—it’s essential. Complete, accurate, and up-to-date identity data is critical for ensuring care quality and patient safety, optimising revenue cycle management, maintaining regulatory compliance, and advancing value-based care. In the public sector, person matching plays a key role in streamlining operations and accelerating benefits delivery. And for any organisation executing AI and ML initiatives, accurate identity data is foundational for generating actionable insights, improving decision-making, and enabling more personalised, efficient care.
Identifying and matching patient, member, and client records is no small task. In the healthcare world, identity data is often scattered across different EHR systems, lab and pharmacy solutions, CRM platforms, and billing, payment, and scheduling apps. In the public sector each program typically maintains its own data independently. Even data from programs within the same agency serving the same clients can remain hopelessly siloed. Name changes, address updates, spelling mistakes, and other discrepancies often lead to duplicate, inconsistent, incomplete, or outdated information.
The cost of getting it wrong is high. Identity data errors and gaps can impact clinical, operational, and business performance, damage your organisation’s reputation, and lead to costly regulatory fines and legal settlements.
- 35% of all denied claims result from inaccurate patient identification or information¹
- The average cost of repeated care because of duplicate records is over $1,700 per ED visit and $1,950 per inpatient stay¹
Enterprise Master Person Indexes Improve Data Accuracy
Enterprise master person indexes² (EMPIs) reconcile patient, member, and client records across disparate systems, eliminating data gaps and inconsistencies. They identify and link records from different sources, providing a uniform, uniquely identified record for each person. They help healthcare organisations streamline M&A integrations and AI initiatives. And they help public sector organisations improve efficiency and coordinate program delivery.
Traditional EMPIs use two different types of algorithms to identify records belonging to the same person.
- Deterministic algorithms look for exact matches of specific data elements like name, date of birth, or social security number. If all criteria match, the records are deemed to belong to the same individual.
- Probabilistic algorithms use statistical analysis to determine the likelihood that two records belong to the same person. They assign a score indicating the probability of a match based on similarities in multiple attributes.
Next-generation EMPIs support advanced algorithms to enhance identity data accuracy and completeness even further.
InterSystems EMPI Solution
InterSystems EMPI™ is a next-generation EMPI that makes it easy to link person records across disparate systems. It integrates seamlessly with InterSystems Unified Care Record, merging identity data from diverse sources into a single, accurate, longitudinal health record.
Key InterSystems EMPI features include:
- A fully customizable linkage engine that uses machine learning and custom rules to automatically match person records
- Comprehensive dashboards and reports for viewing records, analysing data quality, and identifying trends
- Built-in APIs for popular healthcare data standards including HL7 FHIR®, HL7® v2, and IHE
- Choice of on-premises or managed service deployment
More About InterSystems EMPI
Increase Revenue, Reduce Costs, Enhance Experience
Getting provider data right can lead to higher patient satisfaction, increased patient engagement, effective referral management and enhanced operational efficiency.
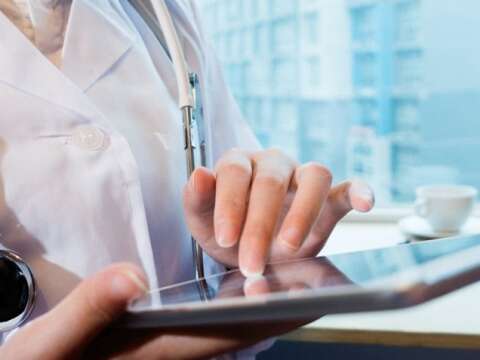
1 - Black Book Market Research
2 - An EMPI is sometimes referred to as Enterprise Master Patient Index