Secure and clean FHIR data can help providers produce valuable insights to improve population health outcomes and personalized care.
The healthcare landscape is awash in data. Electronic health records (EHRs), claims data, patient-generated data — the volume is staggering. But for many hospitals and health systems, extracting meaningful insights from this data remains a challenge due to difficulties finding, accessing, and sharing data. This is where clean and readily accessible data repositories built on the Fast Healthcare Interoperability Resources (FHIR) standard can be game changers. Applying large-scale data technologies such as AI and machine learning to repository data can help improve data quality and normalization, as well as facilitate advanced analytics and insights.
FHIR is a standard for healthcare information exchange developed by Health Level Seven International (HL7) to improve interoperability. FHIR was developed as a free, open standard that brings together the best aspects of prior healthcare formats with modern internet standards.
However, many developers are unfamiliar with FHIR, and increased education on this standard and its capabilities will be crucial to lowering the barrier of entry and easing implementations.
Normalization — organizing and structuring healthcare data — is a foundation of FHIR, making it easier to exchange, understand, and analyze more consistently.
Data is categorized and stored according to corresponding FHIR Resources, the building blocks that define the elements and structure of the exchangeable packets of information according to the standard. A Patient Resource is the demographics for an individual patient, and an Encounter Profile is all the data about a single patient visit (e.g. who, where, why, and when). By focusing on capturing the most essential clinical data elements needed for common healthcare tasks, FHIR makes it easier for systems to exchange core data.
Compared to other healthcare data models, FHIR has a more concise set of data elements. This means there are fewer elements to define, manage, and implement, which can simplify development and integration efforts. By using standardized code sets, FHIR reduces ambiguity and provides consistency in data representation. This makes it easier to understand and interpret the data correctly.
There is also built-in flexibility. While FHIR Resources cover core requirements, healthcare organizations can address specific requirements or use cases by extending or constraining resource definitions via FHIR Profiles. These profiles serve as interoperability rules for specific use cases and help make exchange of FHIR data even more consistent by standardizing the FHIR resources, code sets, and elements. An implementation guide dictates the required constraints for a specific to the use case.
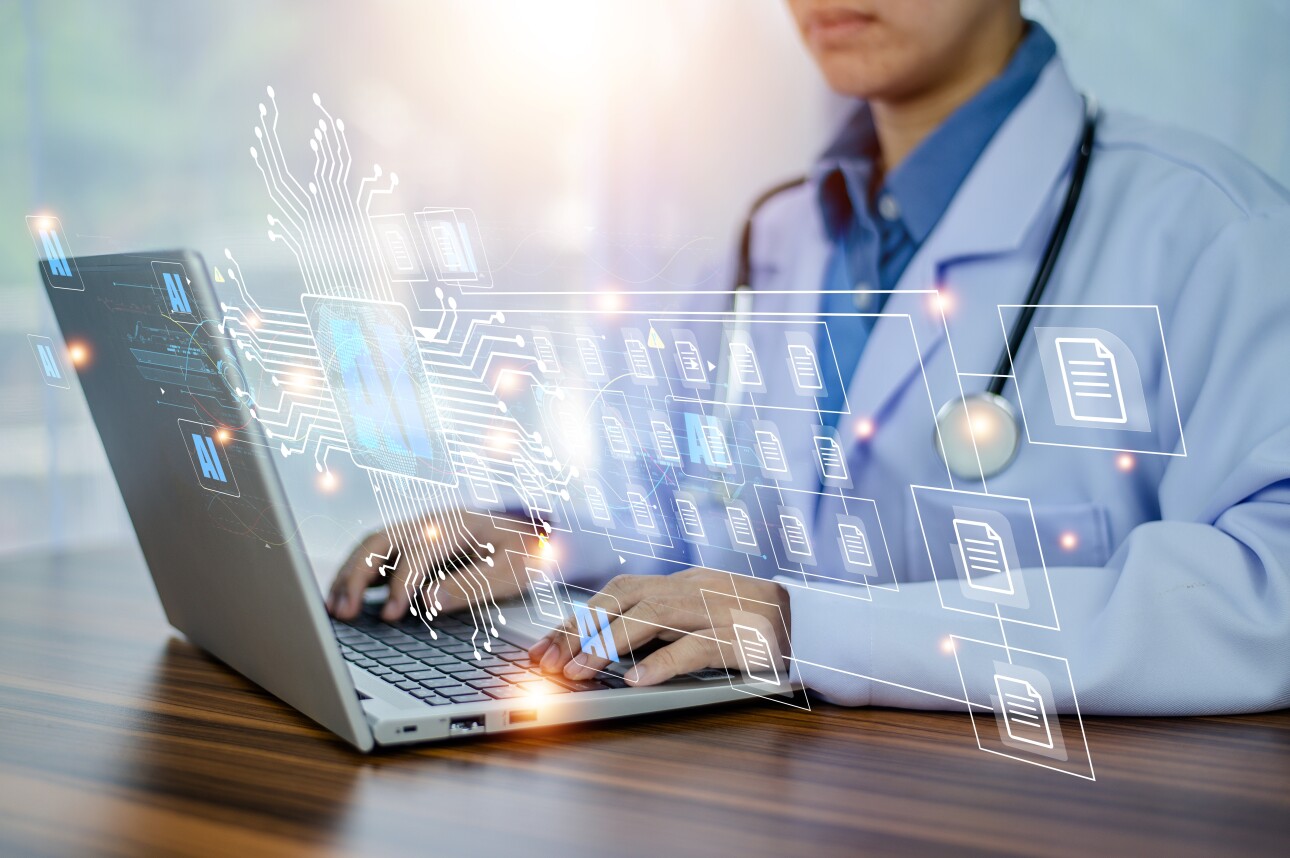
Investing in Big Data Technology for Big Analytics
The first wave of digital health initiatives primarily relied on static data snapshots extracted from disparate systems and integrated into data warehouses. While valuable, these snapshots offer limited understanding of the dynamic nature of healthcare due to several limitations:
- Limited Actionability: Static reports offer limited opportunities for real-time intervention or proactive care management.
- Data Latency: The inherent delay in data extraction and integration leads to outdated insights, hindering timely decision-making.
- Data Silos: Integration challenges persist, creating fragmented views of patient data and hindering holistic analysis.
Digital health leaders are exploring how they can improve healthcare with the increasing amounts of actionable data at their fingertips. One way to increase data-driven healthcare is via expanded FHIR storage and analytics.
FHIR repositories, specialized vaults of FHIR Resource data, hold the key to unlocking the full potential of FHIR in healthcare organizations. A FHIR repository stores FHIR data in its native format so subsequent queries can simply return the FHIR data instead of converting it to FHIR.
To unlock valuable insights from FHIR data, data analysts often use ‘builder’ tools to query FHIR data without having to learn a complex language or query syntax like FHIRPath or FHIR search syntax. This type of analytics allows organizations to improve population health management by identifying trends and patterns in patient populations. Organizations can also analyze patient data for potential health risks and promote early interventions, as well as leverage anonymized patient data for clinical research purposes.
Healthcare organizations also see large-scale data technologies such as AI and machine learning applied to FHIR repositories as a promising way to deliver advanced analytics.
Imagine being able to identify high-risk patients in real-time, trigger-targeted interventions, and monitor their progress continuously. Envision analyzing population-level trends to predict disease outbreaks and proactively allocate resources. With secure, AI-driven FHIR, these scenarios become possible.
While AI algorithms have been applied to EMRs for years, FHIR offers some advantages for AI-driven analytics.
- Data quality and normalization: The consistent and structured nature of normalized data makes it easier to integrate with advanced analytics tools and AI algorithms — AI can automate data cleaning tasks, identifying and correcting inconsistencies and errors, ensuring high-quality data within the repository.
- Real-time, focused analytics: AI can query and analyze specific, relevant FHIR data in real-time for proactive interventions and predict disease outbreaks — tracking patient cohorts, monitoring care quality indicators, and identifying potential issues as they arise.
- Advanced analytics: AI algorithms can analyze massive datasets within the repository, uncovering hidden patterns, predicting health risks, and providing actionable insights for clinicians and researchers.
- Interoperability: FHIR’s standardized format for healthcare data exchange would allow AI to work with data from various systems for a more comprehensive view of a patient’s health.
- Flexibility and reusability: Where AI algorithms designed for one EMR might not be easily adapted to another, FHIR standardization can be applied to data from multiple sources. This reduces development time and may improve collaboration and innovation around AI-driven healthcare analytics.
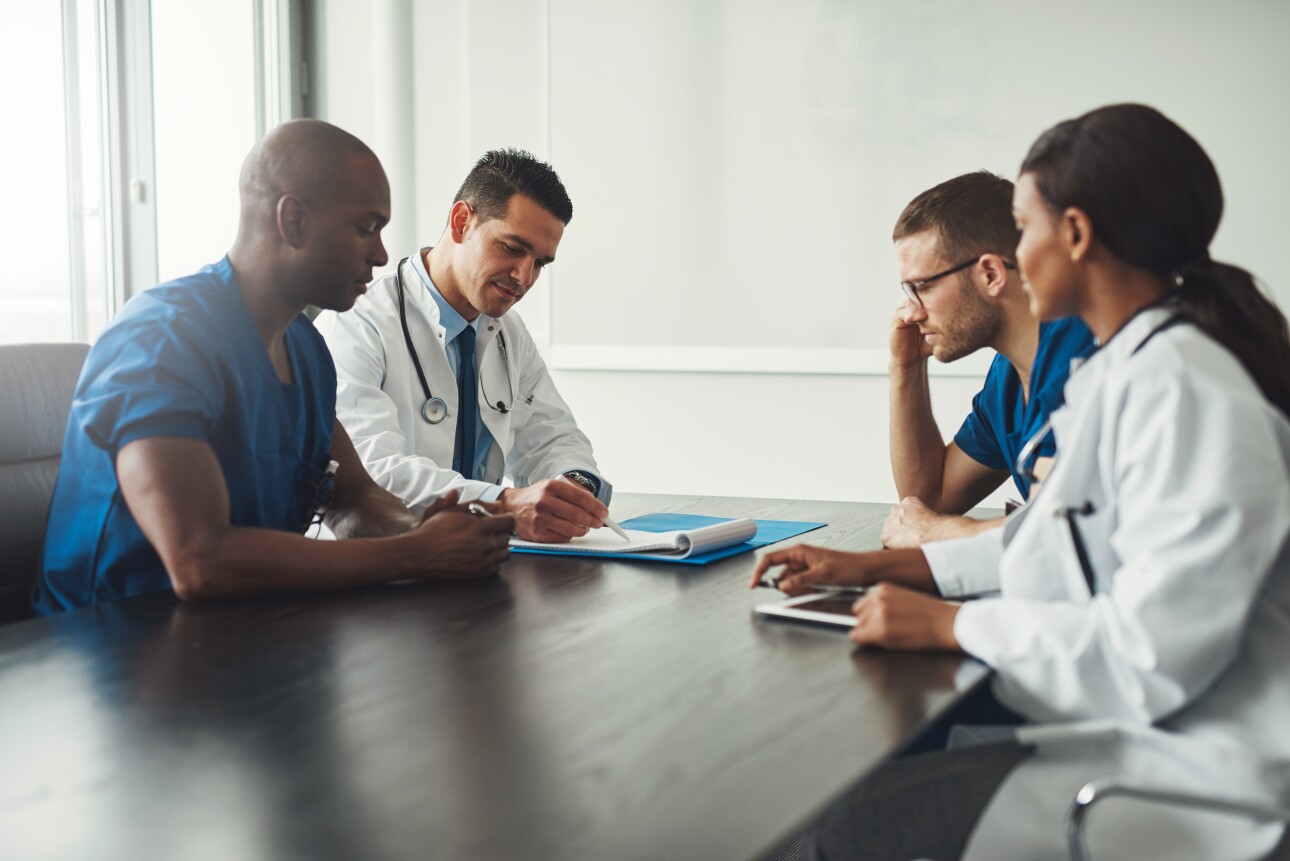
Scaling for the Future: Key Considerations
Scaling your FHIR repository requires careful planning and execution. Here are some key considerations:
- Data quality monitoring: Normalization is an ongoing process, and regularly assessing data quality metrics like completeness, accuracy, consistency, and timeliness can identify areas needing improvement.
- Automation and streamlining: Automation tools, including AI, for data normalization tasks like data mapping, validation, and cleansing can improve efficiency and reduce manual effort.
- Data governance: Establish robust data governance policies to ensure data quality, security, and privacy.
- Benchmarking: Measuring performance at scale is crucial to identify bottlenecks, resource limitations (e.g. memory and storage constraints), and areas for improvements (including volume and demand capabilities), in addition to supporting informed decision-making around repository expansion.
- Security: FHIR repositories house highly sensitive patient information. Robust security measures, like encryption, access controls, and regular audits, are essential to preventing unauthorized access, data breaches, and privacy violations.
- Interoperability: Select technology solutions that offer seamless integration with existing EHRs and other healthcare IT systems.
- Workforce development: Train staff on FHIR standards, AI and other data analysis techniques, and best practices for using the repository.
Building a large-scale, normalized clean data FHIR repository is a strategic investment in the future of healthcare. By embracing big data technology and leveraging the power of secure, AI-driven FHIR, hospital and health system IT leaders can unlock valuable insights, improve population health outcomes, and deliver more personalized and effective care. This journey requires careful planning, collaboration, and investment, but the potential rewards are significant, paving the way for a data-driven future of healthcare.